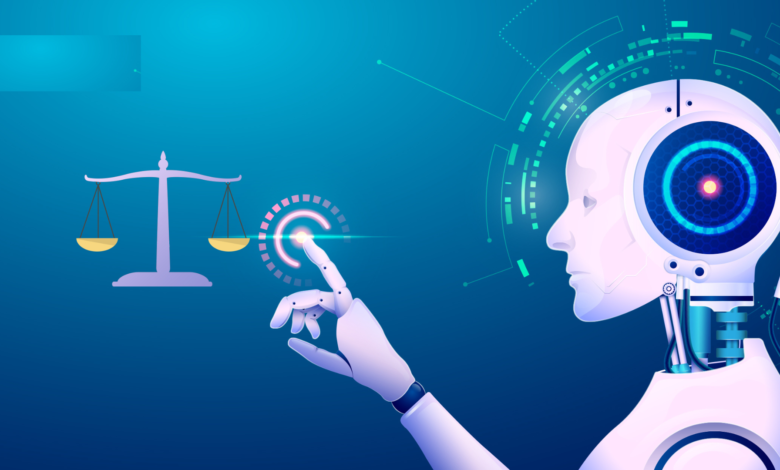
AI Bias Be Avoided: With the advent of AI, the digital world is undergoing rapid transformation. The advent of AI systems has had far-reaching consequences for many industries and occupations, solidifying their place in the technological landscape. The way people engage with technology is one area where artificial intelligence has brought about significant changes. However, other forms of AI bias may have unforeseen effects. Many people are worried about AI becoming too prevalent because of bias.
Artificial intelligence (AI) and machine learning are game-changing technologies that can potentially improve humankind’s future. Meanwhile, it’s critical to lessen the impact of any negative consequences from AI.
Understanding AI Bias
Until recently, AI was primarily a plot point in science fiction stories and movies. But now that far-off idea has become a reality, it has changed many parts of our everyday lives. The healthcare, manufacturing, automotive, and retail sectors are just a few that have seen the immense impact that AI systems have been able to bring about.
There are risks to artificial intelligence that should not be ignored in favor of the benefits of AI systems. You should be aware that a bias in artificial intelligence (AI) guide could help determine how prejudice influences AI performance. Artificial intelligence systems mimic human thought processes, which means they are susceptible to human biases when making decisions. Facial recognition, risk assessment, and chatbot interactions are just a few use cases where AI bias is evident. AI systems that exhibit bias could negatively affect businesses, individuals, and society.
Some other names for AI bias are algorithm bias and machine learning bias. The term “bias in AI systems” describes a situation where decision-making algorithms exhibit systematic mistakes from preconceived assumptions and prejudices. By looking at some examples of AI bias, you can see how it causes judgments to be inaccurate, just like human biases. Artificial intelligence biases occur when AI systems make oversimplified assumptions or employ training datasets that do not portray the target population fairly.
Impact of AI Bias
Understanding the effects of AI bias is the next critical step in eliminating this kind of prejudice. Various effects, from minute shifts to systemic mistakes or erroneous assumptions, can be traced back to AI bias. Consider the possibility that gender bias in society may inspire the creation of AI systems that prefer male applicants over female ones when filling open positions. Conversely, healthcare workers’ unconscious biases toward women of color could pave the way for artificial intelligence systems that misdiagnose women of color.
Research suggests AI face recognition algorithms may misidentify people with darker skin tones more often. According to Stanford University’s Human-Centered AI Institute research, Contrastive Language-Image Pre-training (CLIP) models misclassified persons with dark skin tones.
It exemplifies the influence of racial bias in AI-based face recognition systems and is one of the first instances of AI bias. In this age of growing diversity and representation, it is critical to recognize the potential problems such biases could cause. People from minority groups may experience adverse effects as a result of racial bigotry, for instance.
What are the Popular Types of AI Bias?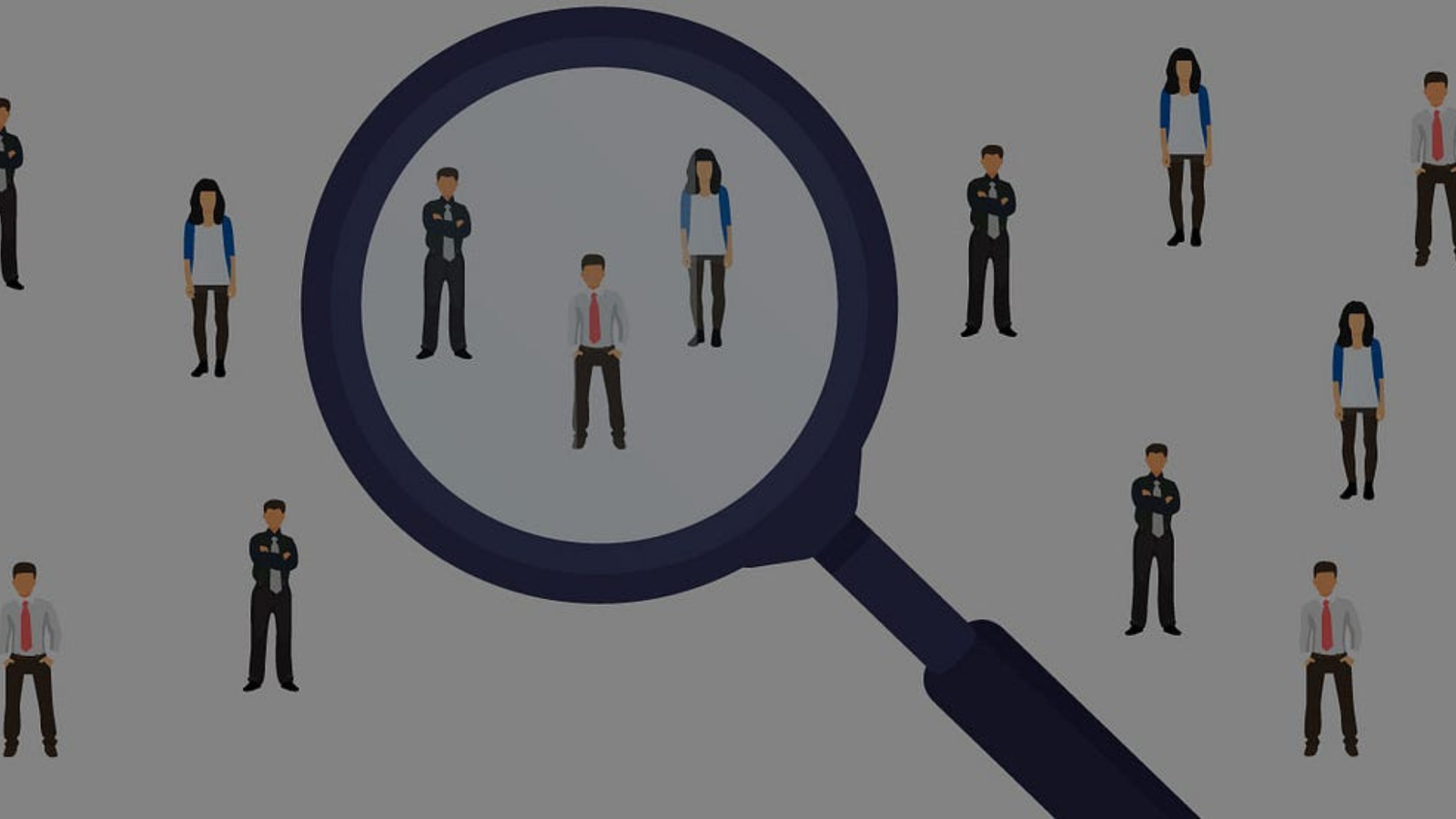
Detecting bias in artificial intelligence may be challenging since it may take many forms. There are two main types of AI bias, algorithmic bias, and data bias, and each has its own set of consequences. Algorithmic bias is the first kind of AI bias. It occurs when AI algorithms produce unfair or erroneous answers because they were trained using faulty assumptions or datasets.
An algorithmic bias may result when biases are present in the data used to train the algorithm. For instance, other populations would be ignored if a training dataset focused on a specific demography. Consequently, the AI algorithm’s output can mirror the dataset’s inherent biases. Consequently, the algorithm’s fairness would be upset.
Data bias is another common suggestion for an AI bias guide. This bias occurs when artificial intelligence (AI) algorithms are trained with datasets containing errors or biases. Data cleansing procedures, data gathering techniques, and inherent biases in the data itself are all potential origins of data bias. An over-representation of one gender or ethnicity in a dataset used to train AI systems to predict consumer behavior is one possible scenario. Artificial intelligence algorithms could produce biased findings that benefit specific groups if data bias were to occur.
How Does AI Bias Affect Different Industries?
Artificial intelligence (AI) has exploded in popularity recently, impacting many facets of people’s daily lives. So, AI needs to be fair and impartial. AI systems have the potential to bring about significant positive change in society. Understanding AI’s benefits and drawbacks is crucial for successfully using AI systems, particularly when considering the many biases. The following is a list of potential sectors that could be impacted by AI bias.
Education
Companies in the education industry use AI to decide which pupils to admit to high schools, junior colleges, and universities. On the other hand, biased decision-making based on AI could unfairly exclude some students from the education industry. An AI system may, for example, employ a gender or racial-biased training dataset. In such a scenario, the AI system can potentially manipulate the admissions process to benefit the affected groups.
Media and Entertainment
What you see on your screen as AI algorithms determines news. Consequently, biased news that does not reflect the population could be prioritized by AI algorithms if such articles lean towards particular biases.
Financial Services
One good strategy to combat AI bias is to examine how technology has changed the finance sector. One way in which AI is transforming the financial services industry is by assisting businesses with decision-making processes related to credit ratings and loan approvals.
Unintended consequences can arise when the algorithm used to make decisions in the financial sector is biased towards certain groups. For instance, an AI program could use a dataset including data from samples with white people to pass loan approvals. Unfair loan rejections for persons with darker skin tones may result from the AI system.
Law Enforcement
Because of inherent flaws in facial recognition algorithms, several forms of AI prejudice may potentially impact the criminal justice system. False positives caused by biased AI in face recognition systems could result in unjustified charges and incarceration. People of color face additional challenges because of the disproportionate number of arrests and incarceration rates associated with this issue.
Famous Instances of AI Bias
The bias associated with AI systems has created multiple issues in the real world. Learning about examples of AI bias and their implications for people and different industries is essential. Here are some notable instances of the concerns emerging from AI bias.
Racism in American Healthcare Systems
In a nation like the US, where systemic racism is a problem, artificial intelligence (AI) has the potential to aid in the fight against health disparities. Underrepresented groups may experience poor performance from AI systems trained on datasets that do not accurately reflect healthcare conditions.
A 2019 study found that AI algorithms used to determine which patients require further medical treatment could be biased. For example, the algorithms gave more weight to white patients than black ones. When calculating healthcare costs, the AI algorithm prioritizes individual healthcare requirements. The algorithm, however, presupposed that historical healthcare spending would shed light on its efficacy.
Hiring Algorithm of Amazon
Amazon has taken advantage of automation to further its interests, significantly influencing e-commerce. The original intent was to use it to rate potential employees. However, the AI algorithm demanded modifications because it was skewed toward women.
After nearly a decade of effort, Amazon’s computer models were able to detect commonalities in applicant submissions and begin evaluating resumes. It was indicative of the male-dominated nature of the sector, that most applicants were men. The AI algorithm penalized Resumes submitted by women after determining that all applications should be from males. Amazon modified the programs so that they are now impartial.
How Can You Resolve Biases in AI?
An increasingly prominent aspect of the AI ecosystem is the need to avoid AI bias. To get the most out of AI systems, you should study the following guidelines for eliminating bias in AI.
Don’t Depend Only on Real-World Data
It could seem like real-world data is a reliable and trustworthy way to train AI algorithms. Also, real-world data should not contain any unintended biases from society or individuals. It would be best not to depend only on real-world data when building datasets to train AI systems. However, you should use both synthetic and real-world data. The accuracy and objectivity of the training dataset would be guaranteed.
Emphasis on Transparency
It is challenging to detect biases in AI algorithms due to their complexity and the fact that a thorough comprehension of the dataset and algorithm’s operation mechanism is required. If you want to know how to spot AI bias, every bias guide will tell you that algorithms can only be fair if they are upfront about it. AI systems need to explain clearly how they make decisions. Furthermore, a company should provide details on how responsible AI systems make judgments.
Human in the Loop
Collaborative efforts between humans and computers are the most effective means of eliminating bias from AI algorithms used in the real world. For example, if a machine cannot resolve the problem, people must step in to help. As a result, the system would establish a never-ending feedback loop, allowing it to learn and improve with each cycle. Consequently, human-in-the-loop has the potential to improve accuracy and safety by guaranteeing datasets are accurate.
Consistent Testing of AI and Machine Learning Models
Regular testing of AI and ML models is another noteworthy strategy for addressing various forms of AI bias. They should be tested often to ensure that AI algorithms avoid bias and are accurate. It would be wise to contrast the AI algorithm’s output with that of human-designed algorithms as well. It can be used to ensure the algorithm is fair when making decisions.
Conclusion
Reviewing several instances of AI bias has demonstrated that AI systems have the potential to enhance and make precise conclusions. It is essential to be aware that AI bias can potentially impact the efficacy of AI systems significantly. Concerns about AI’s effect on practical applications may dampen enthusiasm for the technology.
Nevertheless, a thorough familiarity with the various AI biases and efficient methods for reducing their impact is essential. To ensure AI models are fair, you should employ real-world and synthetic data while training them.